
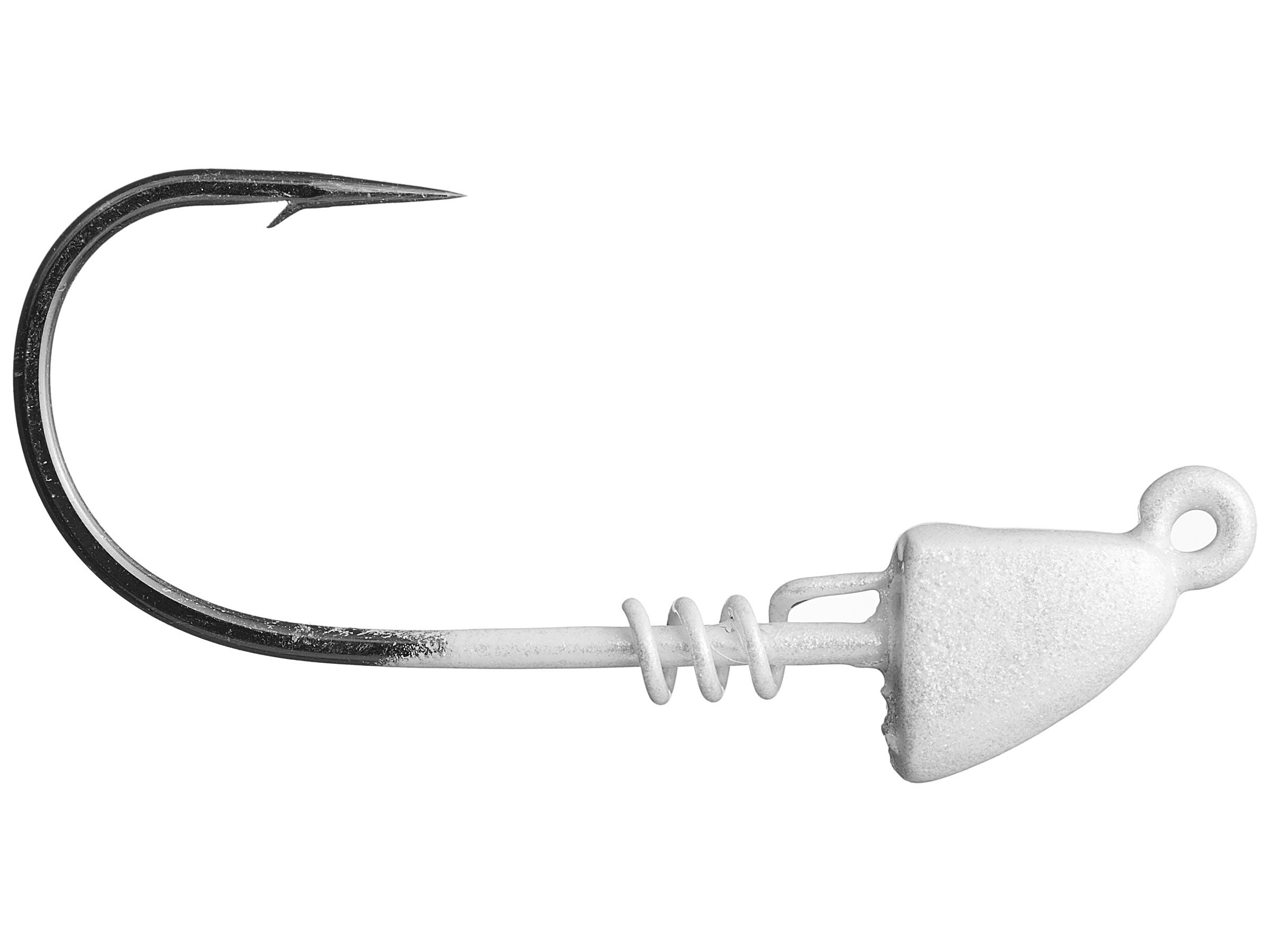
First, before the user-item representations are input into the DNNs, we utilize the attention mechanism to adaptively assign different weights to the user-item representations, which captures the hidden information in implicit feedback. To this end, we propose a deep collaborative recommendation algorithm based on attention mechanism (DACR). Matching function learning-based CF methods directly learn the complicated mapping functions that map user-item pairs to matching scores, which has limitations in identifying low-rank relationships. Representation learning-based collaborative filtering (CF) methods address the linear relationship of user-items with dot products and cannot study the latent nonlinear relationship applied to implicit feedback. Our empirical results on AnimeULike and a standard recommender systems benchmark demonstrate significant performance improvements over previous cold start solutions that do not learn to dynamically represent new users. To evaluate our model and demonstrate a novel application, we present a new dataset for anime recommendations, AnimeULike, containing anonymized interactions between ~13k users and 10k animes, and the accompanying recommendation engine which can exclusively serve guest users. This enables effective generalization to items unseen during training. We propose a graph neural network architecture that inductively learns item and inter-item (edge) representations as a combination of deep language encodings of textual content descriptions and graph embeddings learned via message passing on the edges. Simultaneously, we design a graph representation space, where items (nodes) are connected by edges that signify joint, written recommendations between items. A set of seed items is submitted by the user to use the service, allowing each user to be represented as a function of a collection of items. In this work, we propose new representations for guest users based on their ``content basket’'. However, this process can often be invasive, requiring new users to submit personal data, or shallow, yielding unexpressive representations for accurate recommendations. Prior work addresses this problem by learning profiling user representations to bootstrap recommendations for new users. Guest users are common in real world applications, requiring industrial recommendation systems to handle the "cold start" problem, where no existing interactions between new users and recommendable items can be drawn from to make predictions. Extensive experiments on three real-world datasets from different domains show that CDL can significantly advance the state of the art.


(CF-based) input and propose in this paper a hierarchical Bayesian model called collaborative deep learning (CDL), which jointly performs deep representation learning for the content information and collaborative filtering for the ratings (feedback) matrix. To address this problem, we generalize recent advances in deep learning from i.i.d. Nevertheless, the latent representation learned by CTR may not be very effective when the auxiliary information is very sparse. Collaborative topic regression (CTR) is an appealing recent method taking this approach which tightly couples the two components that learn from two different sources of information. To address this sparsity problem, auxiliary information such as item content information may be utilized. However, the ratings are often very sparse in many applications, causing CF-based methods to degrade significantly in their recommendation performance. Conventional CF-based methods use the ratings given to items by users as the sole source of information for learning to make recommendation. Collaborative filtering (CF) is a successful approach commonly used by many recommender systems.
